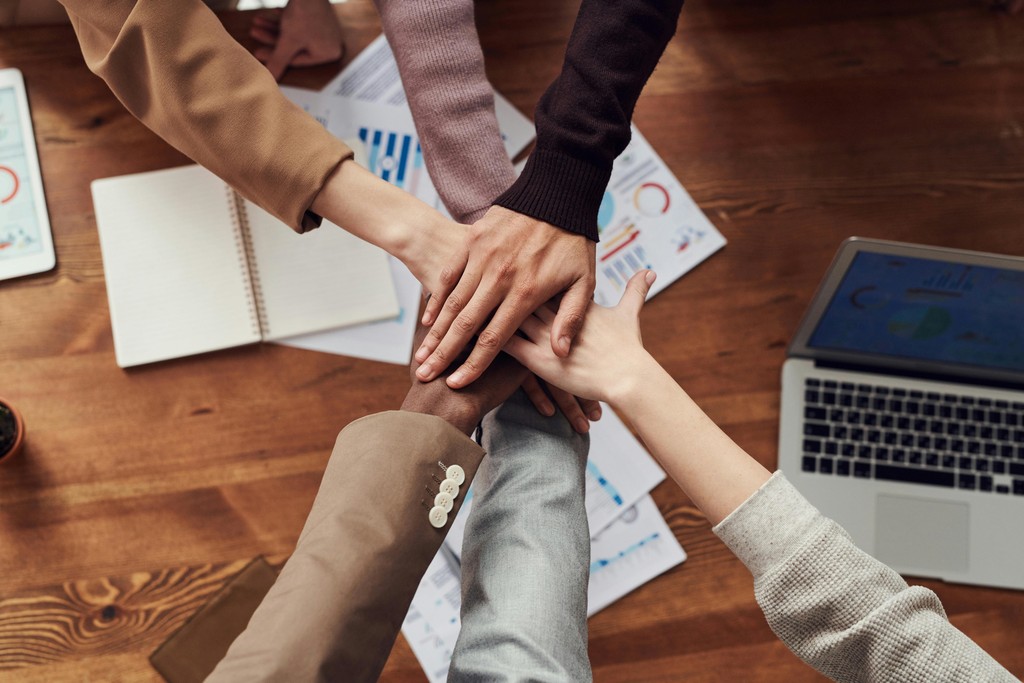
Blog /
Blog /
30 Most Common Linear Regression Interview Questions You Should Prepare For
30 Most Common Linear Regression Interview Questions You Should Prepare For
30 Most Common Linear Regression Interview Questions You Should Prepare For
Mar 18, 2025
Mar 18, 2025
30 Most Common Linear Regression Interview Questions You Should Prepare For
30 Most Common Linear Regression Interview Questions You Should Prepare For
30 Most Common Linear Regression Interview Questions You Should Prepare For
Written by
Written by
Jason Bannis
Jason Bannis
30 Most Common Linear Regression Interview Questions You Should Prepare For
Preparing for a linear regression interview questions interview can be daunting. Mastering common questions not only boosts your confidence but also significantly enhances your performance. This guide provides you with 30 frequently asked linear regression interview questions questions, complete with insights on why they are asked, how to answer them effectively, and example answers to help you ace your interview.
What are linear regression interview questions interview questions?
linear regression interview questions interview questions are designed to assess your understanding of linear regression, a fundamental statistical technique used for modeling the relationship between variables. These questions cover a range of topics, from basic definitions and assumptions to more advanced concepts like model evaluation and regularization. Interviewers use these questions to gauge your analytical skills, your ability to apply theoretical knowledge to practical scenarios, and your problem-solving capabilities in the context of data analysis.
Why do interviewers ask linear regression interview questions questions?
Interviewers ask linear regression interview questions questions to evaluate several key competencies. They want to determine if you:
Understand the core principles of linear regression.
Can identify and address the assumptions underlying linear regression.
Know how to evaluate and interpret linear regression models.
Are capable of handling common issues like multicollinearity and overfitting.
Can communicate complex statistical concepts clearly and concisely.
By asking these questions, interviewers aim to assess your overall proficiency in using linear regression as a tool for data analysis and prediction.
Preview of the 30 linear regression interview questions Interview Questions:
What is Linear Regression?
What are the types of Linear Regression?
What are the equations for Simple Linear Regression (SLR) and Multiple Linear Regression (MLR)?
What are the assumptions of linear regression?
How do you check the assumptions of linear regression?
How do you evaluate the performance of a linear regression model?
How do you interpret coefficients in Multiple Linear Regression?
What if the assumptions of linear regression are violated? How do you address them?
What are the overfitting concerns in Multiple vs Simple Linear Regression Models?
What are Lasso vs Ridge vs Elastic Net Regularization Techniques?
30 linear regression interview questions Interview Questions
What is Linear Regression?
Why you might get asked this: This is a foundational question designed to assess your basic understanding of linear regression. It helps the interviewer gauge your familiarity with the core concepts.
How to answer:
Define linear regression as a statistical method.
Explain its purpose in modeling the relationship between a dependent variable and one or more independent variables.
Mention that it involves fitting a linear equation to observed data.
Example answer:
"Linear regression is a statistical method used to model the relationship between a dependent variable and one or more independent variables by fitting a linear equation to observed data. It helps in predicting the value of the dependent variable based on the values of the independent variables."
What are the types of Linear Regression?
Why you might get asked this: This question tests your knowledge of the different types of linear regression and their applications.
How to answer:
Identify the two main types: Simple Linear Regression (SLR) and Multiple Linear Regression (MLR).
Briefly explain the difference between them.
Example answer:
"There are two main types of linear regression: Simple Linear Regression (SLR), which uses one independent variable, and Multiple Linear Regression (MLR), which uses multiple independent variables to predict the dependent variable."
What are the equations for Simple Linear Regression (SLR) and Multiple Linear Regression (MLR)?
Why you might get asked this: This question assesses your understanding of the mathematical formulation of linear regression models.
How to answer:
Provide the equation for SLR: ( Y = \beta_0 + \beta_1 X ).
Provide the equation for MLR: ( Y = \beta_0 + \beta_1 X_1 + \beta_2 X_2 + ... + \beta_n X_n ).
Explain what each term represents.
Example answer:
"The equation for Simple Linear Regression is ( Y = \beta_0 + \beta_1 X ), where Y is the dependent variable, X is the independent variable, (\beta_0) is the intercept, and (\beta_1) is the slope. For Multiple Linear Regression, the equation is ( Y = \beta_0 + \beta_1 X_1 + \beta_2 X_2 + ... + \beta_n X_n ), where Y is the dependent variable, (X_1, X_2, ..., X_n) are the independent variables, (\beta_0) is the intercept, and (\beta_1, \beta_2, ..., \beta_n) are the coefficients for each independent variable."
What are the assumptions of linear regression?
Why you might get asked this: This question evaluates your understanding of the conditions under which linear regression is valid and reliable.
How to answer:
List the key assumptions: linearity, independence of errors, homoscedasticity (constant variance of errors), and normality of errors.
Briefly explain each assumption.
Example answer:
"The key assumptions of linear regression are: 1) Linearity: the relationship between the variables is linear. 2) Independence: the errors are independent of each other. 3) Homoscedasticity: the variance of the errors is constant across all levels of the independent variables. 4) Normality: the errors are normally distributed."
How do you check the assumptions of linear regression?
Why you might get asked this: This question assesses your ability to verify the validity of linear regression models in practice.
How to answer:
Mention the use of diagnostic plots such as scatter plots, Q-Q plots, and residual plots.
Explain how each plot helps in assessing a specific assumption.
Example answer:
"We can check the assumptions using various diagnostic plots. Scatter plots can help assess linearity, Q-Q plots can check for normality of residuals, and residual plots can help check for homoscedasticity. Additionally, correlation matrices can be used to check for multicollinearity among independent variables."
How do you evaluate the performance of a linear regression model?
Why you might get asked this: This question tests your knowledge of the metrics used to assess the accuracy and reliability of linear regression models.
How to answer:
List relevant metrics such as R-squared (( R^2 )), Mean Squared Error (MSE), and Root Mean Squared Error (RMSE).
Explain what each metric represents and how it is interpreted.
Example answer:
"The performance of a linear regression model can be evaluated using metrics like R-squared (( R^2 )), which represents the proportion of variance in the dependent variable explained by the model; Mean Squared Error (MSE), which measures the average squared difference between the predicted and actual values; and Root Mean Squared Error (RMSE), which is the square root of the MSE and provides a more interpretable measure of the model's accuracy."
How do you interpret coefficients in Multiple Linear Regression?
Why you might get asked this: This question assesses your ability to understand and explain the meaning of the coefficients in a multiple linear regression model.
How to answer:
Explain that each coefficient represents the change in the dependent variable for a one-unit increase in an independent variable, holding all other variables constant.
Emphasize the "holding all other variables constant" aspect.
Example answer:
"In Multiple Linear Regression, each coefficient represents the change in the dependent variable for a one-unit increase in the corresponding independent variable, assuming all other independent variables are held constant. This is crucial for understanding the unique impact of each predictor."
What if the assumptions of linear regression are violated? How do you address them?
Why you might get asked this: This question tests your problem-solving skills and knowledge of techniques to handle violations of linear regression assumptions.
How to answer:
Provide examples of violations and corresponding remedies, such as transformations for non-normal residuals, time-series analysis for autocorrelation, and dimensionality reduction or regularization for multicollinearity.
Example answer:
"If the assumptions are violated, several approaches can be taken. For non-normal residuals, transformations like the Box-Cox transformation can be applied. Autocorrelation might require time-series analysis techniques. Multicollinearity can be handled using dimensionality reduction techniques or regularization methods like Lasso or Ridge regression."
What are the overfitting concerns in Multiple vs Simple Linear Regression Models?
Why you might get asked this: This question assesses your understanding of the risk of overfitting when using more complex models.
How to answer:
Explain that Multiple Linear Regression models are more prone to overfitting due to the increased number of parameters.
Mention that regularization techniques can help mitigate overfitting.
Example answer:
"Multiple Linear Regression models are more susceptible to overfitting than Simple Linear Regression models because they have more parameters. This can lead to the model fitting the noise in the data rather than the underlying relationship. Regularization techniques, such as Lasso and Ridge regression, are often used to mitigate this issue by penalizing large coefficients."
What are Lasso vs Ridge vs Elastic Net Regularization Techniques?
Why you might get asked this: This question tests your knowledge of different regularization methods used to prevent overfitting in linear regression models.
How to answer:
Explain that these methods add penalties to coefficients during optimization.
Describe the differences: Lasso uses L1 regularization (can set coefficients to zero), Ridge uses L2 regularization (reduces but does not eliminate coefficients), and Elastic Net is a combination of both.
Example answer:
"Lasso, Ridge, and Elastic Net are regularization techniques that add penalties to the coefficients during optimization to prevent overfitting. Lasso uses L1 regularization, which can set some coefficients to exactly zero, effectively performing variable selection. Ridge uses L2 regularization, which reduces the magnitude of coefficients but does not eliminate them. Elastic Net combines both L1 and L2 regularization, providing a balance between variable selection and coefficient shrinkage."
Explain the difference between correlation and regression.
Why you might get asked this: This question checks your understanding of the fundamental differences between two related but distinct statistical concepts.
How to answer:
Define correlation as a measure of the strength and direction of a linear relationship between two variables.
Define regression as a method for modeling the relationship between variables to predict outcomes.
Highlight that correlation does not imply causation, while regression can be used for prediction.
Example answer:
"Correlation measures the strength and direction of a linear relationship between two variables, but it doesn't imply causation. Regression, on the other hand, is a method for modeling the relationship between variables to predict outcomes. While correlation can inform regression analysis, regression aims to establish a predictive model."
What is multicollinearity, and how does it affect linear regression models?
Why you might get asked this: This question assesses your understanding of a common issue in multiple linear regression and its implications.
How to answer:
Define multicollinearity as a high correlation between independent variables in a multiple regression model.
Explain that it can lead to unstable coefficient estimates and difficulty in interpreting the individual effects of predictors.
Example answer:
"Multicollinearity occurs when there is a high correlation between independent variables in a multiple regression model. It can lead to unstable coefficient estimates, making it difficult to determine the individual effect of each predictor on the dependent variable. It can also inflate the standard errors of the coefficients, leading to insignificant p-values."
How do you detect multicollinearity?
Why you might get asked this: This question tests your knowledge of methods for identifying multicollinearity in a dataset.
How to answer:
Mention methods like correlation matrices and Variance Inflation Factor (VIF).
Explain how to interpret the results of these methods.
Example answer:
"Multicollinearity can be detected using correlation matrices to identify high correlations between independent variables. Another method is to calculate the Variance Inflation Factor (VIF) for each independent variable. A VIF value greater than 5 or 10 is often considered indicative of significant multicollinearity."
What are some ways to handle multicollinearity?
Why you might get asked this: This question assesses your ability to address multicollinearity and mitigate its effects on a linear regression model.
How to answer:
Suggest methods such as removing one of the correlated variables, combining them into a single variable, or using regularization techniques.
Example answer:
"Multicollinearity can be handled by removing one of the correlated variables from the model, combining the correlated variables into a single variable, or using regularization techniques like Ridge or Lasso regression, which can help stabilize coefficient estimates."
Explain the difference between R-squared and Adjusted R-squared.
Why you might get asked this: This question checks your understanding of how to evaluate the goodness-of-fit of a linear regression model, especially in the context of multiple predictors.
How to answer:
Define R-squared as the proportion of variance in the dependent variable explained by the model.
Explain that Adjusted R-squared adjusts for the number of predictors in the model, penalizing the inclusion of irrelevant variables.
Example answer:
"R-squared represents the proportion of variance in the dependent variable explained by the model. However, it tends to increase as more predictors are added, even if those predictors don't significantly improve the model. Adjusted R-squared adjusts for the number of predictors in the model, penalizing the inclusion of irrelevant variables, and provides a more accurate measure of the model's goodness-of-fit."
What is the purpose of feature scaling in linear regression?
Why you might get asked this: This question assesses your understanding of data preprocessing techniques and their importance in linear regression.
How to answer:
Explain that feature scaling is used to standardize the range of independent variables.
Mention that it can help improve the convergence of optimization algorithms and prevent variables with larger values from dominating the model.
Example answer:
"Feature scaling is used to standardize the range of independent variables. This can help improve the convergence of optimization algorithms, such as gradient descent, and prevent variables with larger values from dominating the model. Common methods include Min-Max scaling and standardization (Z-score scaling)."
Explain the concept of heteroscedasticity and its impact on linear regression.
Why you might get asked this: This question tests your understanding of one of the key assumptions of linear regression and its consequences when violated.
How to answer:
Define heteroscedasticity as the unequal variance of errors across different levels of the independent variables.
Explain that it can lead to inefficient and biased coefficient estimates.
Example answer:
"Heteroscedasticity refers to the unequal variance of errors across different levels of the independent variables. This violates one of the key assumptions of linear regression and can lead to inefficient and biased coefficient estimates, making the model less reliable."
How can you detect heteroscedasticity?
Why you might get asked this: This question assesses your ability to identify heteroscedasticity in a dataset.
How to answer:
Mention methods like visual inspection of residual plots and statistical tests like the Breusch-Pagan test or White's test.
Example answer:
"Heteroscedasticity can be detected by visually inspecting residual plots to see if the variance of the residuals changes systematically across different levels of the independent variables. Statistical tests like the Breusch-Pagan test or White's test can also be used to formally test for heteroscedasticity."
What are some ways to address heteroscedasticity?
Why you might get asked this: This question assesses your knowledge of techniques to handle heteroscedasticity and mitigate its effects on a linear regression model.
How to answer:
Suggest methods such as transforming the dependent variable, using weighted least squares regression, or using heteroscedasticity-consistent standard errors.
Example answer:
"Heteroscedasticity can be addressed by transforming the dependent variable (e.g., using a logarithmic transformation), using weighted least squares regression to give different weights to observations with different error variances, or using heteroscedasticity-consistent standard errors (e.g., White's standard errors) to obtain more reliable inference."
What is the difference between ordinary least squares (OLS) and gradient descent?
Why you might get asked this: This question checks your understanding of different methods for estimating the coefficients in a linear regression model.
How to answer:
Explain that OLS is a closed-form solution that directly calculates the coefficients, while gradient descent is an iterative optimization algorithm that minimizes the cost function.
Example answer:
"Ordinary Least Squares (OLS) is a closed-form solution that directly calculates the coefficients that minimize the sum of squared errors. Gradient descent, on the other hand, is an iterative optimization algorithm that minimizes the cost function by updating the coefficients in small steps. OLS is computationally efficient for small to medium-sized datasets, while gradient descent is often used for large datasets where OLS is computationally infeasible."
What is the significance of the p-value in linear regression?
Why you might get asked this: This question assesses your understanding of statistical significance and hypothesis testing in the context of linear regression.
How to answer:
Explain that the p-value represents the probability of observing the given results (or more extreme results) if the null hypothesis is true.
Mention that a small p-value (typically less than 0.05) indicates strong evidence against the null hypothesis.
Example answer:
"The p-value in linear regression represents the probability of observing the given results (or more extreme results) if the null hypothesis is true. In the context of a coefficient, the null hypothesis is that the coefficient is zero (i.e., the independent variable has no effect on the dependent variable). A small p-value (typically less than 0.05) indicates strong evidence against the null hypothesis, suggesting that the independent variable has a statistically significant effect on the dependent variable."
How do you handle outliers in linear regression?
Why you might get asked this: This question tests your knowledge of how to identify and address outliers, which can disproportionately influence the results of linear regression.
How to answer:
Suggest methods such as identifying outliers using visual inspection or statistical tests, and then either removing them, transforming them, or using robust regression techniques.
Example answer:
"Outliers can be handled by first identifying them using visual inspection of scatter plots or statistical tests like Cook's distance. Once identified, options include removing the outliers, transforming the data to reduce their impact, or using robust regression techniques that are less sensitive to outliers."
What is the purpose of interaction terms in linear regression?
Why you might get asked this: This question assesses your understanding of how to model more complex relationships between variables in linear regression.
How to answer:
Explain that interaction terms are used to model situations where the effect of one independent variable on the dependent variable depends on the value of another independent variable.
Example answer:
"Interaction terms are used to model situations where the effect of one independent variable on the dependent variable depends on the value of another independent variable. For example, the effect of advertising spending on sales might depend on the level of brand awareness. Including an interaction term allows the model to capture these more complex relationships."
Explain the concept of endogeneity and its impact on linear regression.
Why you might get asked this: This question tests your understanding of a more advanced topic related to causality and bias in linear regression.
How to answer:
Define endogeneity as a situation where an independent variable is correlated with the error term in the regression model.
Explain that it can lead to biased and inconsistent coefficient estimates.
Example answer:
"Endogeneity occurs when an independent variable is correlated with the error term in the regression model. This can happen due to omitted variables, measurement error, or simultaneity. Endogeneity leads to biased and inconsistent coefficient estimates, making it difficult to draw valid causal inferences."
How can you address endogeneity in linear regression?
Why you might get asked this: This question assesses your knowledge of techniques to handle endogeneity and obtain unbiased estimates in linear regression.
How to answer:
Suggest methods such as using instrumental variables (IV) regression or two-stage least squares (2SLS) regression.
Example answer:
"Endogeneity can be addressed using techniques like instrumental variables (IV) regression or two-stage least squares (2SLS) regression. These methods involve finding an instrument, which is a variable that is correlated with the endogenous independent variable but not correlated with the error term. The instrument is then used to predict the endogenous variable, and this predicted value is used in the regression model."
What is polynomial regression, and when is it used?
Why you might get asked this: This question checks your understanding of extensions of linear regression that can model non-linear relationships.
How to answer:
Explain that polynomial regression is a form of regression in which the relationship between the independent variable and the dependent variable is modeled as an nth-degree polynomial.
Mention that it is used when the relationship is non-linear but can be approximated by a polynomial function.
Example answer:
"Polynomial regression is a form of regression in which the relationship between the independent variable and the dependent variable is modeled as an nth-degree polynomial. It is used when the relationship is non-linear but can be approximated by a polynomial function. For example, a quadratic relationship can be modeled using a second-degree polynomial."
What are some assumptions of the errors in a linear regression model?
Why you might get asked this: This question aims to dive deeper into the specifics of the assumptions underlying linear regression.
How to answer:
Highlight key assumptions such as that the errors are normally distributed, have a mean of zero, and have constant variance (homoscedasticity). Also, that the errors are independent of each other.
Example answer:
"The errors in a linear regression model are assumed to be normally distributed, have a mean of zero, have constant variance (homoscedasticity), and be independent of each other. Violations of these assumptions can affect the validity of the model and the reliability of the coefficient estimates."
What is the difference between a fixed effect and a random effect in regression models?
Why you might get asked this: This question tests your understanding of more advanced regression models, particularly in panel data settings.
How to answer:
Explain that fixed effects models control for time-invariant characteristics of the entities being observed, while random effects models treat these characteristics as random variables.
Example answer:
"In regression models, particularly in panel data settings, fixed effects models control for time-invariant characteristics of the entities being observed (e.g., individuals, firms, countries) by including separate intercepts for each entity. Random effects models, on the other hand, treat these characteristics as random variables and assume that they are uncorrelated with the independent variables in the model. The choice between fixed effects and random effects depends on the specific research question and the assumptions about the data."
What considerations should guide the choice of variables to include in a linear regression model?
Why you might get asked this: This question assesses your understanding of the principles of model building and variable selection.
How to answer:
Mention considerations such as the theoretical relevance of the variables, their statistical significance, and the potential for multicollinearity.
Example answer:
"The choice of variables to include in a linear regression model should be guided by several considerations, including the theoretical relevance of the variables to the research question, their statistical significance in the model, and the potential for multicollinearity. It's also important to consider the possibility of omitted variable bias and to include control variables to account for potential confounding factors."
How do you validate a linear regression model?
Why you might get asked this: This question tests your understanding of model validation techniques and their importance in ensuring the generalizability of the model.
How to answer:
Suggest methods such as splitting the data into training and testing sets, using cross-validation, and evaluating the model's performance on the testing set.
Example answer:
"A linear regression model can be validated by splitting the data into training and testing sets, fitting the model on the training set, and evaluating its performance on the testing set using metrics like R-squared, MSE, and RMSE. Cross-validation can also be used to obtain a more robust estimate of the model's performance. Additionally, it's important to check the model's assumptions on the testing set to ensure that they are still satisfied."
Other tips to prepare for a linear regression interview questions interview
Review Statistical Concepts: Brush up on basic statistical concepts such as probability, distributions, and hypothesis testing.
Practice with Datasets: Work through practical examples using datasets to apply your knowledge of linear regression.
Understand Assumptions: Thoroughly understand the assumptions of linear regression and how to check them.
Know Evaluation Metrics: Familiarize yourself with common evaluation metrics and their interpretations.
Communicate Clearly: Practice explaining complex concepts in a clear and concise manner.
Stay Updated: Keep abreast of the latest trends and techniques in data analysis and machine learning.
By preparing with these tips and mastering the common linear regression interview questions questions outlined in this guide, you can approach your interview with confidence and increase your chances of success.
Ace Your Interview with Verve AI
Need a boost for your upcoming interviews? Sign up for Verve AI—your all-in-one AI-powered interview partner. With tools like the Interview Copilot, AI Resume Builder, and AI Mock Interview, Verve AI gives you real-time guidance, company-specific scenarios, and smart feedback tailored to your goals. Join thousands of candidates who've used Verve AI to land their dream roles with confidence and ease. 👉 Learn more and get started for free at https://vervecopilot.com/.
FAQ
Q: What is the most important concept to understand for a linear regression interview?
A: Understanding the assumptions of linear regression is crucial. Interviewers often focus on your ability to identify, check, and address violations of these assumptions.
Q: How much statistics do I need to know for a linear regression interview?
A: A solid foundation in basic statistical concepts like probability, distributions, hypothesis testing, and statistical significance is essential.
Q: What are some common mistakes to avoid in a linear regression interview?
A: Avoid neglecting the assumptions of linear regression, failing to address multicollinearity, and not being able to interpret the coefficients and evaluation metrics correctly.
Conclusion
Ready to take your linear regression interview preparation to the next level? Check out our other blog posts and resources on data analysis and machine learning!
30 Most Common Linear Regression Interview Questions You Should Prepare For
Preparing for a linear regression interview questions interview can be daunting. Mastering common questions not only boosts your confidence but also significantly enhances your performance. This guide provides you with 30 frequently asked linear regression interview questions questions, complete with insights on why they are asked, how to answer them effectively, and example answers to help you ace your interview.
What are linear regression interview questions interview questions?
linear regression interview questions interview questions are designed to assess your understanding of linear regression, a fundamental statistical technique used for modeling the relationship between variables. These questions cover a range of topics, from basic definitions and assumptions to more advanced concepts like model evaluation and regularization. Interviewers use these questions to gauge your analytical skills, your ability to apply theoretical knowledge to practical scenarios, and your problem-solving capabilities in the context of data analysis.
Why do interviewers ask linear regression interview questions questions?
Interviewers ask linear regression interview questions questions to evaluate several key competencies. They want to determine if you:
Understand the core principles of linear regression.
Can identify and address the assumptions underlying linear regression.
Know how to evaluate and interpret linear regression models.
Are capable of handling common issues like multicollinearity and overfitting.
Can communicate complex statistical concepts clearly and concisely.
By asking these questions, interviewers aim to assess your overall proficiency in using linear regression as a tool for data analysis and prediction.
Preview of the 30 linear regression interview questions Interview Questions:
What is Linear Regression?
What are the types of Linear Regression?
What are the equations for Simple Linear Regression (SLR) and Multiple Linear Regression (MLR)?
What are the assumptions of linear regression?
How do you check the assumptions of linear regression?
How do you evaluate the performance of a linear regression model?
How do you interpret coefficients in Multiple Linear Regression?
What if the assumptions of linear regression are violated? How do you address them?
What are the overfitting concerns in Multiple vs Simple Linear Regression Models?
What are Lasso vs Ridge vs Elastic Net Regularization Techniques?
30 linear regression interview questions Interview Questions
What is Linear Regression?
Why you might get asked this: This is a foundational question designed to assess your basic understanding of linear regression. It helps the interviewer gauge your familiarity with the core concepts.
How to answer:
Define linear regression as a statistical method.
Explain its purpose in modeling the relationship between a dependent variable and one or more independent variables.
Mention that it involves fitting a linear equation to observed data.
Example answer:
"Linear regression is a statistical method used to model the relationship between a dependent variable and one or more independent variables by fitting a linear equation to observed data. It helps in predicting the value of the dependent variable based on the values of the independent variables."
What are the types of Linear Regression?
Why you might get asked this: This question tests your knowledge of the different types of linear regression and their applications.
How to answer:
Identify the two main types: Simple Linear Regression (SLR) and Multiple Linear Regression (MLR).
Briefly explain the difference between them.
Example answer:
"There are two main types of linear regression: Simple Linear Regression (SLR), which uses one independent variable, and Multiple Linear Regression (MLR), which uses multiple independent variables to predict the dependent variable."
What are the equations for Simple Linear Regression (SLR) and Multiple Linear Regression (MLR)?
Why you might get asked this: This question assesses your understanding of the mathematical formulation of linear regression models.
How to answer:
Provide the equation for SLR: ( Y = \beta_0 + \beta_1 X ).
Provide the equation for MLR: ( Y = \beta_0 + \beta_1 X_1 + \beta_2 X_2 + ... + \beta_n X_n ).
Explain what each term represents.
Example answer:
"The equation for Simple Linear Regression is ( Y = \beta_0 + \beta_1 X ), where Y is the dependent variable, X is the independent variable, (\beta_0) is the intercept, and (\beta_1) is the slope. For Multiple Linear Regression, the equation is ( Y = \beta_0 + \beta_1 X_1 + \beta_2 X_2 + ... + \beta_n X_n ), where Y is the dependent variable, (X_1, X_2, ..., X_n) are the independent variables, (\beta_0) is the intercept, and (\beta_1, \beta_2, ..., \beta_n) are the coefficients for each independent variable."
What are the assumptions of linear regression?
Why you might get asked this: This question evaluates your understanding of the conditions under which linear regression is valid and reliable.
How to answer:
List the key assumptions: linearity, independence of errors, homoscedasticity (constant variance of errors), and normality of errors.
Briefly explain each assumption.
Example answer:
"The key assumptions of linear regression are: 1) Linearity: the relationship between the variables is linear. 2) Independence: the errors are independent of each other. 3) Homoscedasticity: the variance of the errors is constant across all levels of the independent variables. 4) Normality: the errors are normally distributed."
How do you check the assumptions of linear regression?
Why you might get asked this: This question assesses your ability to verify the validity of linear regression models in practice.
How to answer:
Mention the use of diagnostic plots such as scatter plots, Q-Q plots, and residual plots.
Explain how each plot helps in assessing a specific assumption.
Example answer:
"We can check the assumptions using various diagnostic plots. Scatter plots can help assess linearity, Q-Q plots can check for normality of residuals, and residual plots can help check for homoscedasticity. Additionally, correlation matrices can be used to check for multicollinearity among independent variables."
How do you evaluate the performance of a linear regression model?
Why you might get asked this: This question tests your knowledge of the metrics used to assess the accuracy and reliability of linear regression models.
How to answer:
List relevant metrics such as R-squared (( R^2 )), Mean Squared Error (MSE), and Root Mean Squared Error (RMSE).
Explain what each metric represents and how it is interpreted.
Example answer:
"The performance of a linear regression model can be evaluated using metrics like R-squared (( R^2 )), which represents the proportion of variance in the dependent variable explained by the model; Mean Squared Error (MSE), which measures the average squared difference between the predicted and actual values; and Root Mean Squared Error (RMSE), which is the square root of the MSE and provides a more interpretable measure of the model's accuracy."
How do you interpret coefficients in Multiple Linear Regression?
Why you might get asked this: This question assesses your ability to understand and explain the meaning of the coefficients in a multiple linear regression model.
How to answer:
Explain that each coefficient represents the change in the dependent variable for a one-unit increase in an independent variable, holding all other variables constant.
Emphasize the "holding all other variables constant" aspect.
Example answer:
"In Multiple Linear Regression, each coefficient represents the change in the dependent variable for a one-unit increase in the corresponding independent variable, assuming all other independent variables are held constant. This is crucial for understanding the unique impact of each predictor."
What if the assumptions of linear regression are violated? How do you address them?
Why you might get asked this: This question tests your problem-solving skills and knowledge of techniques to handle violations of linear regression assumptions.
How to answer:
Provide examples of violations and corresponding remedies, such as transformations for non-normal residuals, time-series analysis for autocorrelation, and dimensionality reduction or regularization for multicollinearity.
Example answer:
"If the assumptions are violated, several approaches can be taken. For non-normal residuals, transformations like the Box-Cox transformation can be applied. Autocorrelation might require time-series analysis techniques. Multicollinearity can be handled using dimensionality reduction techniques or regularization methods like Lasso or Ridge regression."
What are the overfitting concerns in Multiple vs Simple Linear Regression Models?
Why you might get asked this: This question assesses your understanding of the risk of overfitting when using more complex models.
How to answer:
Explain that Multiple Linear Regression models are more prone to overfitting due to the increased number of parameters.
Mention that regularization techniques can help mitigate overfitting.
Example answer:
"Multiple Linear Regression models are more susceptible to overfitting than Simple Linear Regression models because they have more parameters. This can lead to the model fitting the noise in the data rather than the underlying relationship. Regularization techniques, such as Lasso and Ridge regression, are often used to mitigate this issue by penalizing large coefficients."
What are Lasso vs Ridge vs Elastic Net Regularization Techniques?
Why you might get asked this: This question tests your knowledge of different regularization methods used to prevent overfitting in linear regression models.
How to answer:
Explain that these methods add penalties to coefficients during optimization.
Describe the differences: Lasso uses L1 regularization (can set coefficients to zero), Ridge uses L2 regularization (reduces but does not eliminate coefficients), and Elastic Net is a combination of both.
Example answer:
"Lasso, Ridge, and Elastic Net are regularization techniques that add penalties to the coefficients during optimization to prevent overfitting. Lasso uses L1 regularization, which can set some coefficients to exactly zero, effectively performing variable selection. Ridge uses L2 regularization, which reduces the magnitude of coefficients but does not eliminate them. Elastic Net combines both L1 and L2 regularization, providing a balance between variable selection and coefficient shrinkage."
Explain the difference between correlation and regression.
Why you might get asked this: This question checks your understanding of the fundamental differences between two related but distinct statistical concepts.
How to answer:
Define correlation as a measure of the strength and direction of a linear relationship between two variables.
Define regression as a method for modeling the relationship between variables to predict outcomes.
Highlight that correlation does not imply causation, while regression can be used for prediction.
Example answer:
"Correlation measures the strength and direction of a linear relationship between two variables, but it doesn't imply causation. Regression, on the other hand, is a method for modeling the relationship between variables to predict outcomes. While correlation can inform regression analysis, regression aims to establish a predictive model."
What is multicollinearity, and how does it affect linear regression models?
Why you might get asked this: This question assesses your understanding of a common issue in multiple linear regression and its implications.
How to answer:
Define multicollinearity as a high correlation between independent variables in a multiple regression model.
Explain that it can lead to unstable coefficient estimates and difficulty in interpreting the individual effects of predictors.
Example answer:
"Multicollinearity occurs when there is a high correlation between independent variables in a multiple regression model. It can lead to unstable coefficient estimates, making it difficult to determine the individual effect of each predictor on the dependent variable. It can also inflate the standard errors of the coefficients, leading to insignificant p-values."
How do you detect multicollinearity?
Why you might get asked this: This question tests your knowledge of methods for identifying multicollinearity in a dataset.
How to answer:
Mention methods like correlation matrices and Variance Inflation Factor (VIF).
Explain how to interpret the results of these methods.
Example answer:
"Multicollinearity can be detected using correlation matrices to identify high correlations between independent variables. Another method is to calculate the Variance Inflation Factor (VIF) for each independent variable. A VIF value greater than 5 or 10 is often considered indicative of significant multicollinearity."
What are some ways to handle multicollinearity?
Why you might get asked this: This question assesses your ability to address multicollinearity and mitigate its effects on a linear regression model.
How to answer:
Suggest methods such as removing one of the correlated variables, combining them into a single variable, or using regularization techniques.
Example answer:
"Multicollinearity can be handled by removing one of the correlated variables from the model, combining the correlated variables into a single variable, or using regularization techniques like Ridge or Lasso regression, which can help stabilize coefficient estimates."
Explain the difference between R-squared and Adjusted R-squared.
Why you might get asked this: This question checks your understanding of how to evaluate the goodness-of-fit of a linear regression model, especially in the context of multiple predictors.
How to answer:
Define R-squared as the proportion of variance in the dependent variable explained by the model.
Explain that Adjusted R-squared adjusts for the number of predictors in the model, penalizing the inclusion of irrelevant variables.
Example answer:
"R-squared represents the proportion of variance in the dependent variable explained by the model. However, it tends to increase as more predictors are added, even if those predictors don't significantly improve the model. Adjusted R-squared adjusts for the number of predictors in the model, penalizing the inclusion of irrelevant variables, and provides a more accurate measure of the model's goodness-of-fit."
What is the purpose of feature scaling in linear regression?
Why you might get asked this: This question assesses your understanding of data preprocessing techniques and their importance in linear regression.
How to answer:
Explain that feature scaling is used to standardize the range of independent variables.
Mention that it can help improve the convergence of optimization algorithms and prevent variables with larger values from dominating the model.
Example answer:
"Feature scaling is used to standardize the range of independent variables. This can help improve the convergence of optimization algorithms, such as gradient descent, and prevent variables with larger values from dominating the model. Common methods include Min-Max scaling and standardization (Z-score scaling)."
Explain the concept of heteroscedasticity and its impact on linear regression.
Why you might get asked this: This question tests your understanding of one of the key assumptions of linear regression and its consequences when violated.
How to answer:
Define heteroscedasticity as the unequal variance of errors across different levels of the independent variables.
Explain that it can lead to inefficient and biased coefficient estimates.
Example answer:
"Heteroscedasticity refers to the unequal variance of errors across different levels of the independent variables. This violates one of the key assumptions of linear regression and can lead to inefficient and biased coefficient estimates, making the model less reliable."
How can you detect heteroscedasticity?
Why you might get asked this: This question assesses your ability to identify heteroscedasticity in a dataset.
How to answer:
Mention methods like visual inspection of residual plots and statistical tests like the Breusch-Pagan test or White's test.
Example answer:
"Heteroscedasticity can be detected by visually inspecting residual plots to see if the variance of the residuals changes systematically across different levels of the independent variables. Statistical tests like the Breusch-Pagan test or White's test can also be used to formally test for heteroscedasticity."
What are some ways to address heteroscedasticity?
Why you might get asked this: This question assesses your knowledge of techniques to handle heteroscedasticity and mitigate its effects on a linear regression model.
How to answer:
Suggest methods such as transforming the dependent variable, using weighted least squares regression, or using heteroscedasticity-consistent standard errors.
Example answer:
"Heteroscedasticity can be addressed by transforming the dependent variable (e.g., using a logarithmic transformation), using weighted least squares regression to give different weights to observations with different error variances, or using heteroscedasticity-consistent standard errors (e.g., White's standard errors) to obtain more reliable inference."
What is the difference between ordinary least squares (OLS) and gradient descent?
Why you might get asked this: This question checks your understanding of different methods for estimating the coefficients in a linear regression model.
How to answer:
Explain that OLS is a closed-form solution that directly calculates the coefficients, while gradient descent is an iterative optimization algorithm that minimizes the cost function.
Example answer:
"Ordinary Least Squares (OLS) is a closed-form solution that directly calculates the coefficients that minimize the sum of squared errors. Gradient descent, on the other hand, is an iterative optimization algorithm that minimizes the cost function by updating the coefficients in small steps. OLS is computationally efficient for small to medium-sized datasets, while gradient descent is often used for large datasets where OLS is computationally infeasible."
What is the significance of the p-value in linear regression?
Why you might get asked this: This question assesses your understanding of statistical significance and hypothesis testing in the context of linear regression.
How to answer:
Explain that the p-value represents the probability of observing the given results (or more extreme results) if the null hypothesis is true.
Mention that a small p-value (typically less than 0.05) indicates strong evidence against the null hypothesis.
Example answer:
"The p-value in linear regression represents the probability of observing the given results (or more extreme results) if the null hypothesis is true. In the context of a coefficient, the null hypothesis is that the coefficient is zero (i.e., the independent variable has no effect on the dependent variable). A small p-value (typically less than 0.05) indicates strong evidence against the null hypothesis, suggesting that the independent variable has a statistically significant effect on the dependent variable."
How do you handle outliers in linear regression?
Why you might get asked this: This question tests your knowledge of how to identify and address outliers, which can disproportionately influence the results of linear regression.
How to answer:
Suggest methods such as identifying outliers using visual inspection or statistical tests, and then either removing them, transforming them, or using robust regression techniques.
Example answer:
"Outliers can be handled by first identifying them using visual inspection of scatter plots or statistical tests like Cook's distance. Once identified, options include removing the outliers, transforming the data to reduce their impact, or using robust regression techniques that are less sensitive to outliers."
What is the purpose of interaction terms in linear regression?
Why you might get asked this: This question assesses your understanding of how to model more complex relationships between variables in linear regression.
How to answer:
Explain that interaction terms are used to model situations where the effect of one independent variable on the dependent variable depends on the value of another independent variable.
Example answer:
"Interaction terms are used to model situations where the effect of one independent variable on the dependent variable depends on the value of another independent variable. For example, the effect of advertising spending on sales might depend on the level of brand awareness. Including an interaction term allows the model to capture these more complex relationships."
Explain the concept of endogeneity and its impact on linear regression.
Why you might get asked this: This question tests your understanding of a more advanced topic related to causality and bias in linear regression.
How to answer:
Define endogeneity as a situation where an independent variable is correlated with the error term in the regression model.
Explain that it can lead to biased and inconsistent coefficient estimates.
Example answer:
"Endogeneity occurs when an independent variable is correlated with the error term in the regression model. This can happen due to omitted variables, measurement error, or simultaneity. Endogeneity leads to biased and inconsistent coefficient estimates, making it difficult to draw valid causal inferences."
How can you address endogeneity in linear regression?
Why you might get asked this: This question assesses your knowledge of techniques to handle endogeneity and obtain unbiased estimates in linear regression.
How to answer:
Suggest methods such as using instrumental variables (IV) regression or two-stage least squares (2SLS) regression.
Example answer:
"Endogeneity can be addressed using techniques like instrumental variables (IV) regression or two-stage least squares (2SLS) regression. These methods involve finding an instrument, which is a variable that is correlated with the endogenous independent variable but not correlated with the error term. The instrument is then used to predict the endogenous variable, and this predicted value is used in the regression model."
What is polynomial regression, and when is it used?
Why you might get asked this: This question checks your understanding of extensions of linear regression that can model non-linear relationships.
How to answer:
Explain that polynomial regression is a form of regression in which the relationship between the independent variable and the dependent variable is modeled as an nth-degree polynomial.
Mention that it is used when the relationship is non-linear but can be approximated by a polynomial function.
Example answer:
"Polynomial regression is a form of regression in which the relationship between the independent variable and the dependent variable is modeled as an nth-degree polynomial. It is used when the relationship is non-linear but can be approximated by a polynomial function. For example, a quadratic relationship can be modeled using a second-degree polynomial."
What are some assumptions of the errors in a linear regression model?
Why you might get asked this: This question aims to dive deeper into the specifics of the assumptions underlying linear regression.
How to answer:
Highlight key assumptions such as that the errors are normally distributed, have a mean of zero, and have constant variance (homoscedasticity). Also, that the errors are independent of each other.
Example answer:
"The errors in a linear regression model are assumed to be normally distributed, have a mean of zero, have constant variance (homoscedasticity), and be independent of each other. Violations of these assumptions can affect the validity of the model and the reliability of the coefficient estimates."
What is the difference between a fixed effect and a random effect in regression models?
Why you might get asked this: This question tests your understanding of more advanced regression models, particularly in panel data settings.
How to answer:
Explain that fixed effects models control for time-invariant characteristics of the entities being observed, while random effects models treat these characteristics as random variables.
Example answer:
"In regression models, particularly in panel data settings, fixed effects models control for time-invariant characteristics of the entities being observed (e.g., individuals, firms, countries) by including separate intercepts for each entity. Random effects models, on the other hand, treat these characteristics as random variables and assume that they are uncorrelated with the independent variables in the model. The choice between fixed effects and random effects depends on the specific research question and the assumptions about the data."
What considerations should guide the choice of variables to include in a linear regression model?
Why you might get asked this: This question assesses your understanding of the principles of model building and variable selection.
How to answer:
Mention considerations such as the theoretical relevance of the variables, their statistical significance, and the potential for multicollinearity.
Example answer:
"The choice of variables to include in a linear regression model should be guided by several considerations, including the theoretical relevance of the variables to the research question, their statistical significance in the model, and the potential for multicollinearity. It's also important to consider the possibility of omitted variable bias and to include control variables to account for potential confounding factors."
How do you validate a linear regression model?
Why you might get asked this: This question tests your understanding of model validation techniques and their importance in ensuring the generalizability of the model.
How to answer:
Suggest methods such as splitting the data into training and testing sets, using cross-validation, and evaluating the model's performance on the testing set.
Example answer:
"A linear regression model can be validated by splitting the data into training and testing sets, fitting the model on the training set, and evaluating its performance on the testing set using metrics like R-squared, MSE, and RMSE. Cross-validation can also be used to obtain a more robust estimate of the model's performance. Additionally, it's important to check the model's assumptions on the testing set to ensure that they are still satisfied."
Other tips to prepare for a linear regression interview questions interview
Review Statistical Concepts: Brush up on basic statistical concepts such as probability, distributions, and hypothesis testing.
Practice with Datasets: Work through practical examples using datasets to apply your knowledge of linear regression.
Understand Assumptions: Thoroughly understand the assumptions of linear regression and how to check them.
Know Evaluation Metrics: Familiarize yourself with common evaluation metrics and their interpretations.
Communicate Clearly: Practice explaining complex concepts in a clear and concise manner.
Stay Updated: Keep abreast of the latest trends and techniques in data analysis and machine learning.
By preparing with these tips and mastering the common linear regression interview questions questions outlined in this guide, you can approach your interview with confidence and increase your chances of success.
Ace Your Interview with Verve AI
Need a boost for your upcoming interviews? Sign up for Verve AI—your all-in-one AI-powered interview partner. With tools like the Interview Copilot, AI Resume Builder, and AI Mock Interview, Verve AI gives you real-time guidance, company-specific scenarios, and smart feedback tailored to your goals. Join thousands of candidates who've used Verve AI to land their dream roles with confidence and ease. 👉 Learn more and get started for free at https://vervecopilot.com/.
FAQ
Q: What is the most important concept to understand for a linear regression interview?
A: Understanding the assumptions of linear regression is crucial. Interviewers often focus on your ability to identify, check, and address violations of these assumptions.
Q: How much statistics do I need to know for a linear regression interview?
A: A solid foundation in basic statistical concepts like probability, distributions, hypothesis testing, and statistical significance is essential.
Q: What are some common mistakes to avoid in a linear regression interview?
A: Avoid neglecting the assumptions of linear regression, failing to address multicollinearity, and not being able to interpret the coefficients and evaluation metrics correctly.
Conclusion
Ready to take your linear regression interview preparation to the next level? Check out our other blog posts and resources on data analysis and machine learning!
30 Most Common WordPress Interview Questions and Answers You Should Prepare For
MORE ARTICLES
MORE ARTICLES
MORE ARTICLES
Apr 11, 2025
Apr 11, 2025
Apr 11, 2025
30 Most Common mechanical fresher interview questions You Should Prepare For
30 Most Common mechanical fresher interview questions You Should Prepare For
Apr 7, 2025
Apr 7, 2025
Apr 7, 2025
30 Most Common WPF Interview Questions You Should Prepare For
30 Most Common WPF Interview Questions You Should Prepare For
Apr 11, 2025
Apr 11, 2025
Apr 11, 2025
30 Most Common Java Coding Interview Questions for 5 Years Experience
30 Most Common Java Coding Interview Questions for 5 Years Experience
Ace Your Next Interview with Real-Time AI Support
Ace Your Next Interview with Real-Time AI Support
Ace Your Next Interview with Real-Time AI Support
Get real-time support and personalized guidance to ace live interviews with confidence.
Get real-time support and personalized guidance to ace live interviews with confidence.
Get real-time support and personalized guidance to ace live interviews with confidence.
Try Real-Time AI Interview Support
Try Real-Time AI Interview Support
Try Real-Time AI Interview Support
Click below to start your tour to experience next-generation interview hack